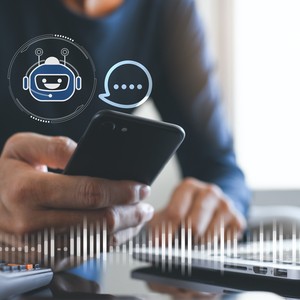
Deep Learning: Not all AI is as Smart
Artificial Intelligence (AI) is possibly the most significant technological advance in recent history. Sadly, it’s also the most abused.
by Tim Knight, CMO, CloudApps.
Many vendors in the software market today are claiming to have an AI-driven Sales solution poised to deliver significant revenue benefits. But simplistic “Deal Insight” recommendations does not an AI-engine make.
We’re looking at you, Salesforce Einstein.
In this post, we demystify some common misconceptions around AI, prove the solid business value behind Deep Learning and call out some reasons why vendors really don’t want buyers to know the difference between the two approaches.
The good news is that AI does, in fact, have the power to fully transform and enhance the way we approach selling today. For the better.
However, in order to get the best out of the machine, we need to first take into consideration a few of its challenges.
Challenge #1: Not all AI is equal
Firstly, let’s think about the term ‘AI’ simply as a label, much in the same way ‘iPhone’ is. In the case of AI, it’s a label that has been given to a discipline of computing rather than a consumer device.
Now consider for a moment that you were in the market to purchase a device labelled ‘iPhone’. You probably wouldn’t settle on purchasing just any old ‘iPhone’. It would be important for you to understand which version of the technology you were buying. After all, there are stark differences in capability between and iPhone v1 and the most recent iPhone v11.
The same is true of AI. However, for buyers of ‘AI’ labelled technology, there is no helpful version label available. Without it, all things branded AI appear equal. And that simply couldn’t be further from the truth.
Challenge #2: It turns out that training the machine is really hard
Computers, like humans, learn by example through a process called Training.
This typically involves supplying numerous examples of the things we would like the computer to learn. And therein lies the first challenge.
If a human was being taught to recognise different shapes, visual senses would be enough to understand the characteristics of the different shapes. For example, we might first consider the number of sides a shape has and then use the angles between sides to categorise it further.
Computer systems obviously don’t possess this ability. Instead, they need to be told which characteristics they should consider in the learning process. This manual process is called Feature Extraction.
Recognising the characteristics of shapes is a relatively straightforward exercise. But imagine how complex this process becomes for non-trivial problems.
This is where data scientists are required. They are the clever folks responsible for Feature Extraction.
Whilst not insurmountable, this approach has meant that traditional Machine Learning is limited to the dexterity of the data scientist and the quality of Feature Extraction performed.
It also means that, regardless of how many examples are provided to the machine, it will eventually hit a ceiling on the level of accuracy it can achieve.
Challenge #3: Most modern AI is actually 50 years old
Meaning that many (if not all) of the currently available AI solutions still follow this rather dated method. Including those from leading vendors such as Einstein from Salesforce.
Let’s contrast that for a moment again to the iPhone. The first iPhone was released in June 2007, less than thirteen years ago. The advances in technology in that time alone have been staggering.
It’s a little bewildering to think that pretty much all of the current AI offerings are based on technology that is approaching fifty years old.
Known as ‘Deep Learning’, this new approach outperforms traditional Machine Learning by huge margins.
What’s the difference? Without diving into the technical detail, Deep Learning has introduced the ability for the machine to determine for itself what features are important. Alleviating the huge burden of Feature Extraction and the expense of Data Scientists.
Unlike the 50-year-old veteran approach to Machine Learning, Deep Learning actually benefits from extensive amounts of data. Learning from it and becoming even more accurate over time.
You might be less surprised to learn that the emergence of this new approach has left the old veteran standing still. Given the step-change in its accuracy, every ounce of research effort is being poured into improving these Deep Learning algorithms. The old model has simply run out of steam and has rightly been put out to grass.
To put this into context we have seen solutions based on the old (let’s call it ‘Shallow Learning’) approach to AI reach 75-80% accuracy. Not bad you might think.
In contrast, however, we have witnessed Deep Learning applied to the same problem achieve over 95% accuracy. Quite some difference and it will only get even sharper over time.
Why is Deep Learning grabbing the headlines and outperforming traditional AI? Because it’s:
- Far more accurate – and only performs better with the more data it’s fed
- Fast to get started – it doesn’t require the processing of vast volumes of data before producing results
- Self-learning – it will continuously learn as your business and buyers habits evolve
- Future-proofed – and will continue to see innovation investment where traditional AI will not
- Learns from time-sequenced data – delivering even great levels of accuracy by taking time & sequence in account
Picking up the final point from the list above, there is another very important difference provided by Deep Learning.
These modern, state-of-the-art algorithms not only recognise patterns in data, they crucially also take time & sequence of that data into account.
This is in direct contrast to traditional (Shallow Learning) algorithms. These have no notion of order in time, and only consider the current example they have been exposed to. This type of AI approach is somewhat of an amnesiac with regard to the past. Not very helpful in a sales situation where both ‘timing’ and ‘what has gone before’ is very often crucial.
No, seriously, we’re better.
Why? Because CloudApps is the first and only provider of ‘Deep Learning’ powered AI in the CRM sector.
Trust comes from reliability.
The CloudApps AI engine is unique in its ability to generate forecasts that are over 95% accurate.
How is it so accurate? Because the CloudApps AI engine:
- Learns from rich behavioural data: More data delivers greater accuracy. We generate a rich data audit trail for every deal based on high-value sales behaviours, not simple sales activity.
- Uncovers from the deal journey: Timing is everything. The data picture we build is time-sequenced, recording not just which sales behaviour happened but crucially when.
- Uses the latest innovation: Not all AI is as smart. We use the very latest ‘Deep Learning’ algorithms that significantly outperform traditional AI.
Our Deep Learning AI engine delivers unprecedented levels of accuracy. Powering our customers to achieve results that include:
- 95% forecast accuracy
- 60% more selling time
- 20% increase in win rate
All with a level of accuracy you can trust.
Why not challenge us to show you the power of the CloudApps Sales Effectiveness Platform by booking your very own live demo.